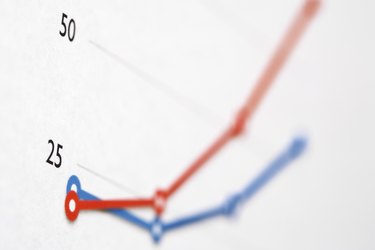
A one-way ANalysis Of VAriance, or ANOVA, is a statistical method used to compare the means of more than two sets of data, to see if they are statistically different from each other. SPSS, a statistical analysis package, allows the use of a one-way ANOVA in its large suite of procedures. However, the ANOVA is not a perfect test and under certain circumstances will provide misleading results.
Sample Limitations
Video of the Day
The ANOVA test assumes that the samples used in the analysis are "Simple random samples." This means that a sample of individuals (data points) are taken from a larger population (a larger data pool). The samples must also be independent -- that is, they do not affect each other. ANOVA is generally suitable for comparing means in controlled studies, but when the samples are not independent a repeated measures test must be used.
Video of the Day
Normal Distribution
ANOVA assumes that the data in the groups are normally distributed. The test can still be carried out should this not be the case -- and if the violation of this assumption is only moderate, the test is still suitable. However, if the data is a long way from the normal distribution, the test will not provide accurate results. To get around this, either transform the data with the SPSS "Compute" function before running the analysis, or use an alternative test such as a Kruskal-Wallace test.
Equal Standard Deviations
Another limitation of ANOVA is that it assumes that the groups have the same, or very similar, standard deviations. The greater the difference in standard deviations between groups, the greater chance that the conclusion of the test is inaccurate. Like the normal distribution assumption, this is not a problem as long as the standard deviations are not hugely different, and the sample sizes of each group are roughly equal. If this is not the case, a Welch test is a better option.
Multiple Comparisons
When you run an ANOVA in SPSS, the resulting F value and significance level only tell you whether at least one group in your analysis is different from at least one other. It does not tell you how many groups, or which groups, differ statistically. In order to determine this, follow-up comparisons must be performed. This is rarely a problem in small analyses, but the higher the number of groups included in the follow-up test, the greater the chance of making a Type I error, which is assuming an effect where there isn't one.